Good afternoon. Yesterday, soon-to-be-public Coinbase reported Q1 earnings. The cryptocurrency exchange made a profit of $730–$800 million on ~$1.8 billion in revenue.
So, yeah...if you think you had a good quarter in crypto, just remember Coinbase’s was better.
In today’s edition:
AI language models
China’s digital yuan
Digital X-rays
—Ryan Duffy, Hayden Field
|
|
Francis Scialabba
As a loyal reader of this newsletter, you probably know that large language models (LLMs) are machine learning algorithms that underpin powerful tools, like predictive texting and Google Search. You might also know that they’ve sparked a fierce debate in recent months.
Recap: Because these pattern-tracking models are trained on large swaths of the internet, they can learn and amplify harmful behaviors, like generating racist and sexist language. Their use has tremendous environmental costs, and their decisions are difficult to track and explain.
Meet the alternatives
Alternative approaches to large language models are slowly gaining traction in research communities, but they haven’t really caught on yet industry-wide. They center on smaller systems—which can do some of the same work that larger models do, but in a more explainable and computationally efficient way.
First to bat: Moderate, retrieval-based language models. Unlike large language models, they keep a lot of the information they need in external storage, so it’s not adding a constant computational burden.
- Think of it like regularly checking out a reference book from the library instead of trying to memorize all the contents, says Gadi Singer, a VP at Intel Labs.
Next up: AI-augmented versions of rule-based models. Since rule-based models are more static, ML can flag areas that need new rules—and write those rules itself. The model’s decisions can then be tied back to the rules it’s using to make them, which helps with explainability.
Switch hitter: Then there’s the tinyML movement, which focuses on small, simple models. The perks: They’re cheaper, can run on more devices, and have strongly reduced power consumption.
- “You can get a model that’s...maybe one one-thousandth the size of a general-purpose LLM, that can focus on one task and do it just as well,” says Leon Derczynski, a machine learning and language scientist.
There’s always a “but”
The data that retrieval-based models keep around for explainability purposes still takes up space. And rules written by a machine learning model are just as susceptible to bias.
Big picture: “I think those models should be thought of as being in the same class, with the same risks,” Dr. Jacob Andreas, assistant professor at MIT, told us. “It’s easier to mitigate them—easier to understand, when a model starts behaving in a bad way, why it happened—but that’s not something fundamentally different.”
Check out the on-site version of this story for more detail on these alternatives. —HF
|
|
Francis Scialabba
For now, central bank digital currencies (CBDCs) are side projects for dozens of governments. Like any good side-hustler, the governments that have made the most progress are making a lot of noise about it.
China’s digital yuan comes to mind. Most recently, the People’s Bank of China began testing the currency in Hong Kong and exploring cross-border transfer protocols with Thailand and the UAE. Digital yuan, known as Digital Currency Electronic Payment (DC/EP), is controlled by a central authority and administered through commercial banks.
DC/EP’s expeditious rollout is driven by China’s robust fintech sector and bitcoin. After studying the cryptocurrency, Beijing’s top banking official was equal parts “dazzled” and “frightened,” per the WSJ.
- The digital yuan is trackable from the top, though officials say some anonymity is built in.
- Digital yuan could become a financial workaround for any company or regime that is persona non grata in Washington.
- China could set expiration dates for digital yuan, inducing citizens or companies to spend it more quickly, the WSJ reports.
Zoom out: Are the other central banks kicking the can down the road? Well, the US would tell you that first ≠ best. —RD
|
|
SPONSORED BY J.P. MORGAN WEALTH MANAGEMENT
|
If you told us we only had three words to describe J.P. Morgan Wealth Management, that’s what we’d say. So much wisdom.
Why? Because J.P. Morgan has spent over 200 years helping investors navigate every type of market.
And that know-how is now available at your fingertips when you trade with $0 commissions on the Chase Mobile app – ranked #1 in customer satisfaction for wealth management apps by J.D. Power.
You’ll get unlimited access to timely insights, education, and research – all designed to help you make smarter investing decisions.
Learn more about investing online with J.P. Morgan Wealth Management here.
J.P. Morgan Securities LLC, Member FINRA/SIPC
|
|
Francis Scialabba
Five years ago, Geoffrey Hinton—AKA the “godfather of neural networks”—said, “People should stop training radiologists now,” because image perception algorithms would soon put them out of work entirely.
That prediction met the same bitter end as forecasts for Quibi subscribers and the long-term value of Beanie Babies. Radiologists are not only employed, but in high demand. They are getting better tech, though.
On Friday, Nano-X Imaging got 510(k) clearance—a significant green light from the FDA—for its digital X-ray technology, which could bring down machine costs.
Why this is big: X-ray tech is pricey and bulky, and it hasn’t changed much in the past century. Right now, machines have to heat cathodes to a high enough temperature to produce electrons and create an X-ray, then cool down those components.
- Nano-X’s approach → building specially designed semiconductors for X-ray production, without all the temperature requirements. The company told The Motley Fool it can mass-produce full-body scanners for $10,000, while current machines can cost 10x that or more.
But, but, but: Nano-X still has to get FDA clearance for its cloud-based software, which allows for direct upload of X-ray images and uses ML-powered diagnostic algorithms to analyze them. —HF
|
|
Francis Scialabba
Stat: According to records reviewed by BuzzFeed News, 7,000+ individuals from nearly 2,000 public agencies nationwide have used Clearview AI to search millions of Americans’ faces.
Quote: "The idea of a centralized social network is just not going to exist five or 10 years in the future. There's a membrane or a chasm between the old world and this new crypto-native universe.”—Tyler Winklevoss to Forbes, when asked about Facebook.
Listen: Tim Cook on the NYT’s Sway podcast. Two choice excerpts: “I can’t talk about anything that may or may not be in the pipeline,” but AR is an important part of the future, Cook confirmed. On cars: “There’s lots of things you can do with autonomy. And we’ll see what Apple does.”
SOC 2 compliance, uncomplicated: Secureframe helps companies get SOC 2 compliant within weeks (rather than months). They monitor 40+ services—including AWS, GCP, and Azure—and save customers 50% on their audit costs. Schedule a demo today.*
*This is sponsored advertising content.
|
|
-
Encryption is an “essential” part of the internet, WhatsApp head Will Cathcart writes in Wired.
-
GM announced that it will make the EV Chevrolet Silverado pickup at a new assembly plant under construction in Detroit. The automaker’s stock closed at an all-time high yesterday.
-
Signal, a WhatsApp rival, is beta-testing a partnership with anonymity-oriented cryptocurrency MobileCoin.
-
NASA successfully deployed the Ingenuity helicopter on Mars.
-
TikTok will add auto-captions for US and Japanese users “in the coming months.”
-
Sarcos, a startup that develops robotic exoskeletons, is planning a SPAC.
|
|
Look at your phone as happily as that person^. Get your access today to income-generation through Yieldstreet. Yieldstreet allows you to invest in unique alternative asset classes with low stock market correlation for as low as $1,000 and target annual yields of 7-15%. And get this, Emerging Tech Brew readers can get $25 for signing up today. Create your Yieldstreet account here.
|
|
Yahoo! Answers will shut down on May 4 for good. It’s the latest erasure of a time capsule from the early Internet 2.0 days, and a reminder that some parts of the web aren’t as permanent as we assume. On that note, let’s hope the links between NFTs and their digital media files’ addresses don’t break.
Anywho, today’s trivia looks at the history of Yahoo!: Take the quiz here.
|
|
A new DARPA-funded dataset—called “Oops!” and made up of 20,338 YouTube fail videos—will help machines learn to flag unintentional human actions. We’re looking forward to the algorithm’s thoughts on that jogger who slipped on the snow and took a hard fall on live TV.
|
|
Catch up on the top Emerging Tech Brew stories from the past few editions:
|
|
Enjoying the newsletter? Share it with your network to take advantage of our rewards program.
When you reach 5 referrals, we'll send you this Emerging Tech Brew sticker sheet.
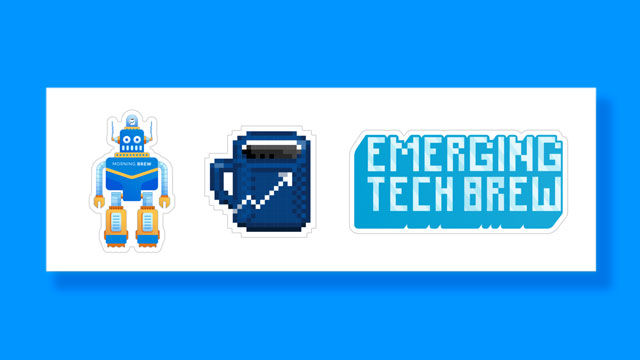
Hit the button below to learn more and access your rewards hub.
Click to ShareOr copy & paste your referral link to others: morningbrew.com/emerging-tech/r/?kid=303a04a9
|
|
Written by
Hayden Field and Ryan Duffy
Was this email forwarded to you? Sign up here.
|
ADVERTISE // CAREERS // SHOP
Update your email preferences or unsubscribe here.
View our privacy policy here.
Copyright © 2021 Morning Brew. All rights reserved.
22 W 19th St, 8th Floor, New York, NY 10011
|
|