Sponsored by | 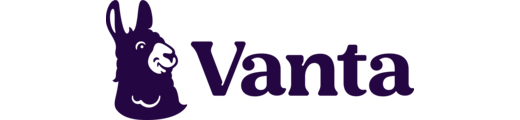 |
| | The next year will be exceptional: the pace of innovation has never been so fast and multidirectional. | Recently, we reached out to you asking to share what your expectations are for 2024. Though making predictions is hard, people love this game, and today’s newsletter is lengthy. For your convenience, we have categorized the replies: jump to those that might be most interesting to you! | These responses are varied, coming from specialists with different perspectives. We recommend reading them all as a whole, to get a wider picture. So buckle up and get ready for 2024. | Table of Contents | | Scaling Your Compliance Program: Free Guide | | Do you need to add more security frameworks to your existing compliance program, but don’t know where to start? Scaling your compliance program can feel like you’re proving your security from scratch. It doesn’t have to. | Vanta’s ultimate guide to scaling your compliance program shares strategies and best practices for adding compliance standards without adding to your workload. | Learn how to scale, manage, and optimize alongside your business goals. | | | From Researchers | Yoshua Bengio*: AI Safety Priorities for 2024 | Massive moonshot and short-term investments in R&D to make progress on AI alignment and evaluations of capabilities, current and anticipated harms and longer-term risks; answers need to arrive and be implemented by regulators before the advances in capabilities create significant dangers (the timeline for which is highly uncertain but trends are worrisome). This requires both national and multilateral efforts as well as incentives for AI developers through regulatory requirements. Setting up global principles for the democratic and multilateral governance and oversight of Frontier AI labs to avoid unfettered concentration of AI-driven power and protect democracies from a major increase in AI-driven disinformation campaigns, especially as 4 billion people on earth are expected to be sent to the polls in 2024. This includes the development of regulation and treaties as well as oversight mechanisms giving a voice not just to the national government of the AI Frontier lab but also civil society, independent academics and representatives of the international community. Setting up government-funded AI Frontier Labs with a non-profit mission and democratic and multilateral governance (as per (2)), in order to help achieve UN SDGs not addressed by commercial AI efforts and protect the public from national security risks and mitigate other potentially catastrophic outcomes with future AI systems harboring malicious goals (set by nefarious humans or of AIs whose control would have been lost).
| *Yoshua Bengio, a Canadian AI pioneer, is renowned for his deep learning advancements. He, with Geoffrey Hinton and Yann LeCun, received the 2018 Turing Award for contributions to neural networks and ML, significantly impacting computer vision, speech recognition, and NLP.
| Turing Post offers the best analysis of where AI comes from, its current impact, and where we are driving it. Please support us and → | |
|
| Sara Hooker, Cohere’s VP Research, Head of Cohere For AI | In 2024, we need to do a better job making sure our technology serves everyone. The development of models and the training datasets have been heavily biased towards English-speaking and Western European countries, offering little representation of languages from the Global South or Asia, where much of the world’s population lives. I predict with projects like Cohere for AI’s AYA LLMs will vastly improve in terms of breadth of languages use to train models, significantly democratizing the technology. 2024 will also be a year for research bets. Multi-modal will become a ubiquitous term as we move away from sub-fields dedicated to language, computer vision and audio in isolation. Models will become more akin to our human intelligence – able to process multiple sensory inputs at once. It will also be the year we focus on the size of models – looking for efficiency rather than size – as it becomes critical to deploy models that are available in resource-constrained environments. Our lab is already pushing the limits of efficiency at scale and adaptive computing (both data pruning and mixture of experts). Finally, 2024 should also be a year when we examine the defaults of where, how and by whom research is done. To date, state of art models have come from a handful of labs and researchers. We will begin to make critical strides and begin to close the significant gaps with regard to who participates in research. In 2024, I hope we create better incentive structures for broadening of participants and cultural backgrounds deeply involved in AI research.
| Vincenzo Manzoni, Data Science Director at Tenaris | | From VC | S. Somasegar, Managing Director at Madrona Venture Group | Rise of small language models (SLMs). Doesn’t mean that the large language models are going away. The world needs LLMs. The world also needs SLMs. Particularly for task specific or domain specific capabilities, I see a world of large and small language models and applications using a combination of these models to optimize price, performance and accuracy as appropriate. Hype around AI though will still be there, is going to be a little more grounded. There is still going to be a lot of excitement around AI. Everybody is going to be thinking about how they leverage AI in whatever they are doing. At the same time, if you are a builder you are going to be more focusing on use cases and business models that are sustainable. If you are a customer, you are going to be thinking about “show me the money” or “what is the ROI” for me? If you are an investor you are going to want to see business model, traction and monetization to go hand in hand with technology innovation. Nvidia is the industry’s best friend (except maybe for Intel, AMD and a few others). Whether you are large technology company or a hyperscale cloud provider or a startup, Nvidia is here to help with chips, hardware, software, cash (largest AI investor in 2023). It is both an amazingly enviable position to be in and a super responsible position to be in to continue driving innovation and staying ahead of the curve.
| Apoorva Pandhi, Managing Director at Zetta VP | Apoorva was working on his 10 predictions for 2024, when I reached out, so I’m choosing a few that resonates the most. You can read the rest here. | LLMs Reimagine the Data Stack - We believe that LLMs will meaningfully transform the data stack. These transformative models will elevate the efficiency of diverse data professionals by fostering collaboration and automating tasks that were traditionally manual—particularly those demanding considerable effort, domain expertise, and time. Beyond serving as copilots for routine tasks, LLMs will unlock innovative capabilities, such as Data Spelunking (disambiguating data, semantic similarity, entity resolution), validating unstructured data quality, and optimizing queries, among other advancements. New Forms of Feedback (RL’X’F) on the Block - In 2023, Reinforcement Learning from Human Feedback (RLHF) has proven surprisingly effective for aligning models towards general human preferences. However, RLHF is expensive, time-consuming and difficult to scale in specialized domains. We expect to see new forms of interactive feedback emerge for domain-specific tasks and non-sensory data like RL (Reinforcement Learning) through AI Feedback, RL through Experimental Feedback, RL through Expert Feedback and more. We will see a new wave of startups address these challenges for enterprises training and fine-tuning their models. RAG evolves from Semantic Similarity to more sophisticated approaches - Though semantic similarity is the current consensus-based approach, it doesn’t work optimally for all RAG use cases. The approach will evolve from semantic similarity towards ontology-based representations (e.g. graph networks) over the next 12-24 months. For example, representing a code base in a graph ontology captures the relationships between embedded and/or interconnected functions in a way that cosine similarity likely doesn’t
| From infrastructure builders | Max Hjelm, VP of Sales at CoreWeave | Will the GPU craze continue? We’re in the first inning of a very long and exciting game. The demand profile for GPU hardware is growing so fast, it will far outpace anything we expect today. Compute requirements to train cutting-edge AI are doubling every 5-6 months and I think that over the next several years, the degree to which it is going to become part of our everyday life hasn't even been explored yet. As Jensen Huang said, we’re seeing two simultaneous platform shifts at the same time – accelerated computing and generative AI. The world is moving away from general purpose computing, the one-size-fits-all infrastructure for the broadest range of use cases. This new era of generative AI demands upgraded data centers and specialized cloud providers. Industries like AI, VFX, and life sciences need a modern, specialized cloud, purpose-built for GPU-intensive workloads. A modern software stack will also prove to be the differentiator for the next generation of cloud companies, to support the most complex and intensive workloads, like generative AI, with a product that's more efficient and more performant at scale compared to legacy platforms.
| Mike Del Balso, Founder and CEO of Tecton | AI will go real time. As product teams look to make their products smarter with AI, they will build experiences that make smart decisions based on the information the customer is providing right now or other real-time context. Think of real-time personalized recommendations, surge pricing, ETAs, fraud detection, player matching in gaming, etc. Being able to do real-time AI will be more important than ever. Infrastructure that supports making this easier (like Tecton) will be very helpful along the way.
| | From Responsible AI specialists | Antonia Bierwirth, Responsible Innovation Manager at Tecnalia Research & Innovation | AI will become more and more human, in the sense that it will be able to understand and support humans in a more holistic way, not just information and analysis. In this way it will take also more human roles, in business but also in our private life. This doesn’t necessary mean human “replacement”, although the issue is questionable, but rather a fruitful collaboration, AI will be a comfortable buddy and partner (more than a tool), to create unimaginable solutions thanks to its increasing divergent thinking and creativity. In the coming year AI will become a successful therapist and coach, combining data with human wisdom and good practices. Talking about trends on a macro level I would definitely mention algocracy.
| Subho Majumdar, Advocate and Practitioner of Responsible AI, Head of AI at Vijil | Responsible AI will become a first order concern in companies. Until now the RAI community hasn't been able to make a case for themselves in industry teams, leading to most such teams being nixed. But the advent of generative AI is going to change that. People are realizing that now when we say AI we're really talking about intelligent software. And you can't say that security, privacy, or non-toxicity is not important in software. In 2024, AI developers are going to realize that they don't have tools, best practices, and standards that help them build responsibly. There are going to be community-driven efforts to bridge that gap. The cybersecurity community is going to take a leading role in such initiatives for providing practical, pragmatic approaches to responsible AI development.
| Misc | Ricardo Acuña, the Principal of Psycognet | LMMs per Domain, Industry and Culture - In 2024 we will see a surge of specialized, domain specific and cultural-aware LMMs. The current LMMs are knowledge generalists from a business, industry and culture domain standpoint. The cloud infrastructure and the abundant offer of commercial and open source LMMs and tools (Azure, AWS, Google) released in 2023, offer an opportunity to develop with speed and ease tailored AI solutions in 2024. LMM Context Management improvement - 2024 will see the arrival of more efficient new and creative techniques and tools that surpass the actual limitations of prompt engineering and fine-tunning methods. The key success factor will be to have a more and better cost/effective and technology efficient LMM context and thought management. Cognitive Psychology techniques - This prediction is more personal biased. Although still in its infancy in academic research, the use of cognitive psychology (and computational psychology) techniques into the LMM frameworks, to resemble or to get closer to a real AGI, would provide a promising alternative to enhance the actual LMM models.
| Antonin Delobre, a student at ESSEC, a business school in France and EU | I think that AI community will face the first big data leakage, this leak will open the door to different solutions more open-source and with more transparency. As well as for web3 economy, the ecological cost of AI will be a major point in 2024, improvements in less energy-intensive LLMs will be a key for AI sustainability. The AI for all will become a very important subject and this will require accessibility to the greatest number,( unfortunately not everyone is able to use Hugging Face)
| Ollie Carmichael, a Product Manager at Adarga Limited | An LLM trained predominately on Mandarin text will rival GPT4 in performance. A medium sized European country will demonstrate how to effectively integrate generative AI into the running of public services and the good conduct of government. The larger NATO nations will continue to focus on how to apply AI to weapons systems, but not focus on building efficiencies within their large bureaucracies. The first large scale GenAI induced redundancies will be reported in the media. This will be a big story, but as ever, the reality will be quite distinct from the media narrative.
| | |
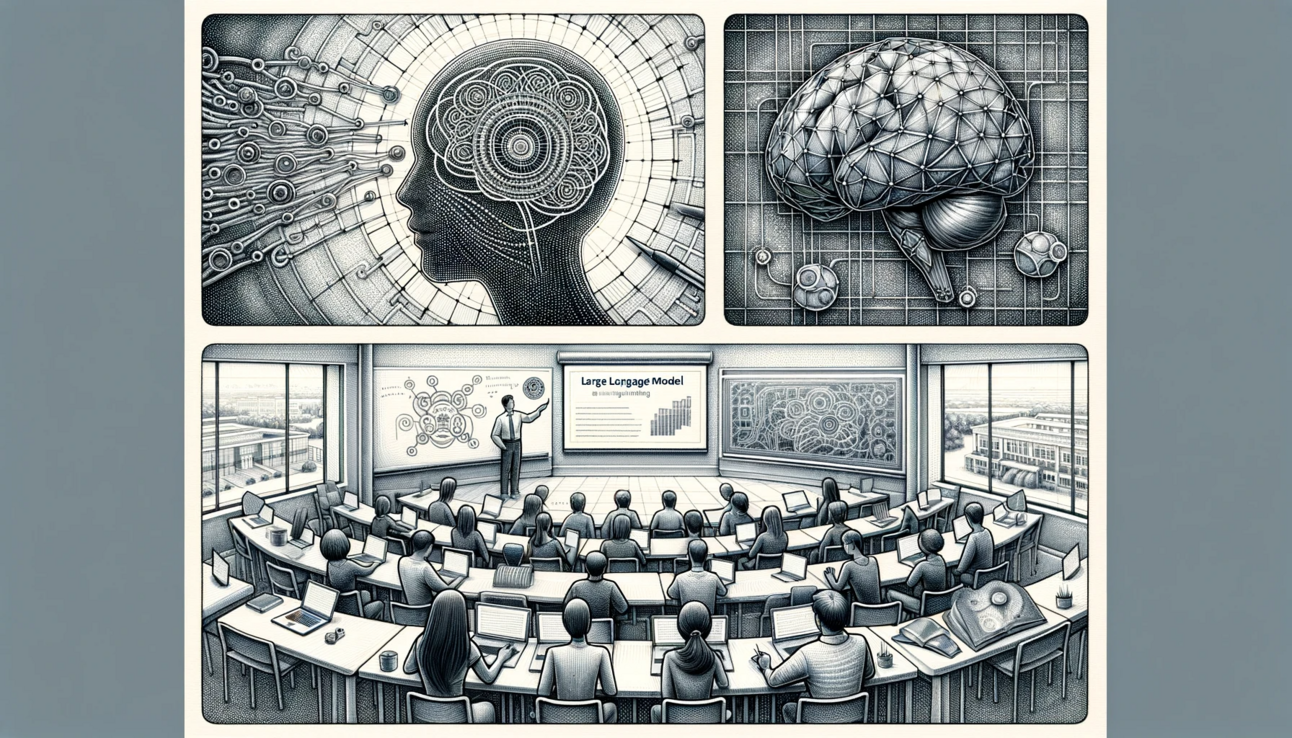 | 8 Free Courses to Master Large Language Models | These courses that will help you to learn more about LLMs – our curated list of best free sources | www.turingpost.com/p/llms-courses |
| 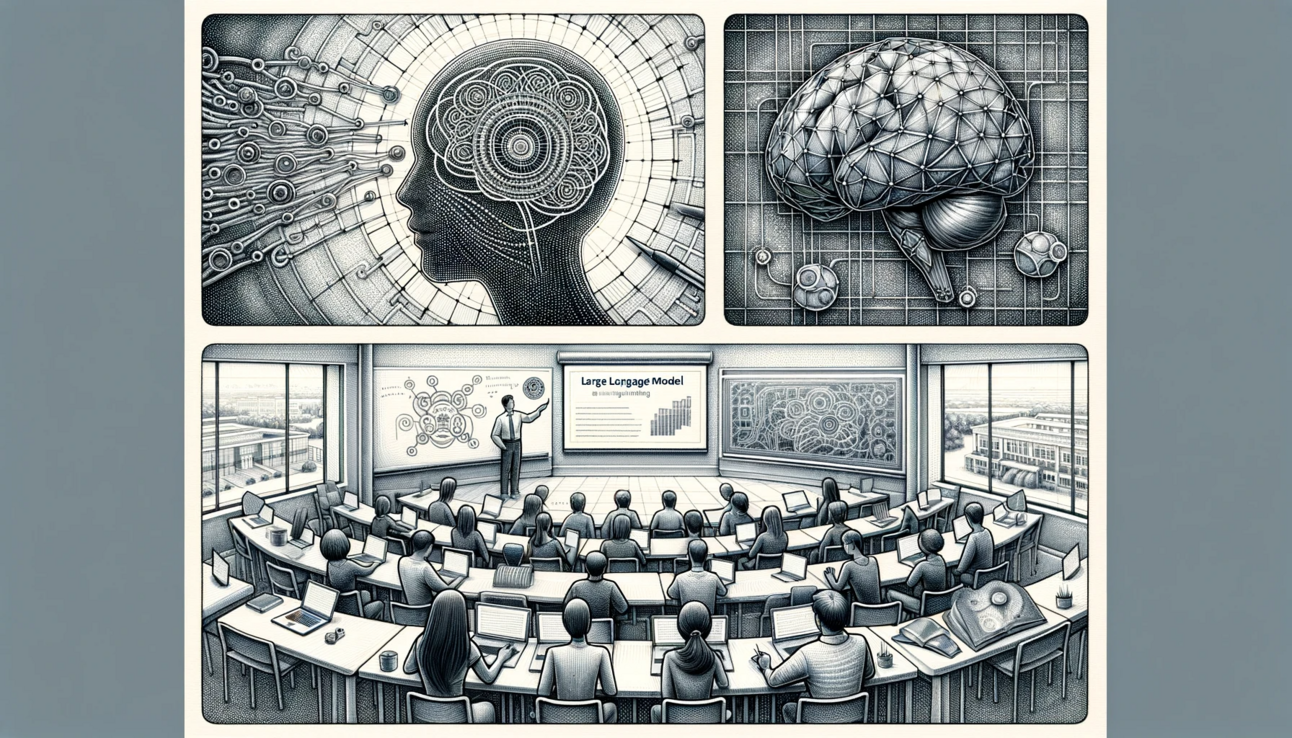 |
|
| | Other news, categorized for your convenience | An exceptionally rich week! As always, we offer you only the freshest and the most relevant research papers of the week. Truly, the best curated selection: | Enhancements in LLM Techniques and Efficiency | Papers focusing on methods and frameworks to improve the training, inference, and efficiency of LLMs: | Weight Subcloning: Efficient training of smaller transformer models. Paper EE-LLM: Early-exit capabilities for efficient LLM training and deployment. Paper SPARQ ATTENTION: Technique for reducing memory bandwidth in LLM inference. Paper SwitchHead: Enhancing Transformers with MoE attention. Paper TinyGSM: Effectiveness of small LLMs in complex problem-solving. Paper Phi-2: Demonstrating the capabilities of a small language model. Paper
| LLM Alignment, Honesty, and Safety | Papers addressing the alignment of LLMs with human intentions, honesty, and safety in AI interactions: | Alignment for Honesty: Framework for honesty alignment in LLMs. Paper Llama Guard: Safeguarding human-AI interactions. Paper Self-Evaluation Improves Selective Generation in LLMs: Enhancing LLMs' response reliability. Paper Steering Llama 2 via Contrastive Activation Addition: Controlling LLM outputs through activation modification. Paper
| AI Applications and Dataset Creation | Exploring the applications of LLMs in various fields and the creation of datasets for specialized tasks: | Faithful Persona-based Conversational Dataset Generation: Improving AI chatbots with persona-based datasets. Paper ReST meets ReAct: Self-improvement in multi-step reasoning for LLMs. Paper VILA: Enhancing visual tasks in LLMs. Paper Honeybee: Projector for multimodal LLMs. Paper Evaluation of LLMs for Autonomous Driving: Decision-making accuracy in autonomous driving. Paper "I Want It That Way": Combining LLMs with Constraint Programming for decision support. Paper
| LLM Evaluation, Open Source, and Future Directions | Evaluating LLMs, promoting open-source models, and exploring future directions and challenges in AI: | LLM360: Advocating for open-source LLMs. Paper PromptBench: A library for LLM evaluation. Paper Foundation Models in Robotics: Applying foundation models in robotics. Paper Challenges with unsupervised LLM knowledge discovery: Critical examination of unsupervised methods in LLMs. Paper Beyond Human Data: Scaling self-training for LLMs. Paper Weak-to-Strong Generalization: Aligning AI models surpassing human capabilities. Paper Context Tuning for Retrieval Augmented Generation: Enhancing RAG in LLMs. Paper Mathematical Language Models: A Survey: Overview of LMs. Survey
|  | TuringPost @TheTuringPost | 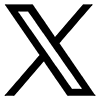 |
| |
NeurIPS is one of the most significant gatherings for the AI/ML communities. We made a list of research papers from 10 companies that we found online: - Amazon - Google - IBM - NVIDIA ... If you know more, please share links in the comments! 🧵 | 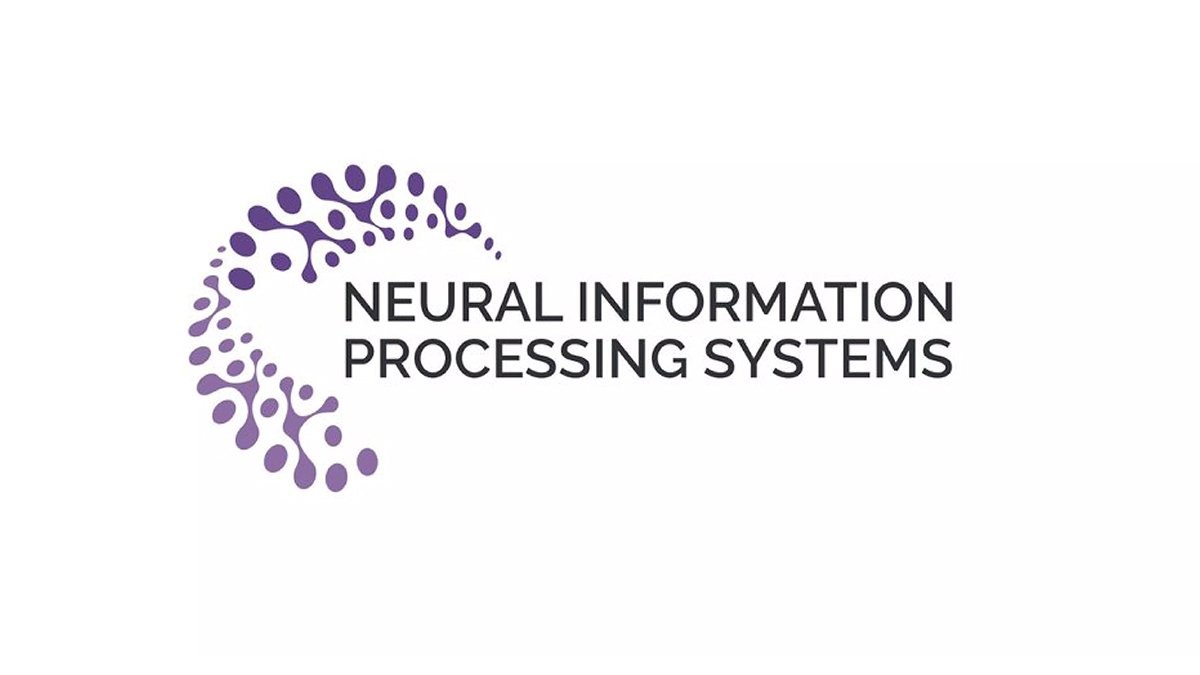 | | Dec 15, 2023 | |  | | 37 Likes 6 Retweets 2 Replies |
|
| Thank you for reading, please feel free to share with your friends and colleagues. In the next couple of weeks, we are announcing our referral program 🤍 | | Another week with fascinating innovations! We call this overview “Froth on the Daydream" - or simply, FOD. It’s a reference to the surrealistic and experimental novel by Boris Vian – after all, AI is experimental and feels quite surrealistic, and a lot of writing on this topic is just a froth on the daydream. | How was today's FOD?Please give us some constructive feedback | | | |
|