|
|
This Week in Turing Post: |
Wednesday, AI 101: a deep dive Hybrid RAG Friday, Agentic Workflows: Introduction of the new series
|
Turing Post is a reader-supported publication, please consider becoming a paid subscriber. You get full access to all our articles, investigations, and tech series immediately → | |
|
|
The main topic |
On-Device AI = mind-blowing mass adaption |
For the first time in years, the new iPhone offers something that truly outshines all other smartphones. Skillfully navigating the turbulent waters of AI, Apple has embraced the best technology available – without burning billions. And because the iPhone is always either in your pocket or setting the competitive pace for other smartphones, on-device AI is truly becoming a reality. In a year, no one will remember a time when we lived without AI in our phones – and here, the "AI" stands for Apple Intelligence. |
Deep Integration of Hardware and AI |
What sets Apple Intelligence apart from generic AI integrations on other platforms is its deep coupling with the silicon. The A18 chip, built on second-gen 3nm technology, not only runs inference tasks but integrates Apple's entire ecosystem. It’s optimized to ensure smooth, real-time applications of AI features like natural language understanding, image generation, and personal context-driven suggestions – all while maintaining energy efficiency, a crucial factor in edge computing. |
A18 Chip: Optimized for AI at the Edge |
Apple’s move with the A18 chip signals a huge leap in on-device AI performance. With a 16-core neural engine optimized for running large-scale generative models, the iPhone 16 is essentially bringing AI to your pocket. For AI professionals, this means a potential playground for running inference tasks on the fly, leveraging optimized hardware without needing cloud resources. The device boasts a 30% faster CPU and a 40% faster GPU compared to its predecessor, iPhone 15. These improvements aren’t just for gaming or photo processing, though – Apple Intelligence aims to embed generative AI into everyday user interactions. |
According to FT, he A18 chip is built on Arm's V9 architecture, a cutting-edge design that Apple has embraced through its multi-year licensing agreement with the UK-based, SoftBank-owned company. Arm's V9 provides the building blocks for the chip’s neural engine, allowing Apple to optimize performance for generative AI workloads directly on-device. This partnership is crucial as Apple leans further into AI, ensuring that its hardware can meet the growing computational demands without compromising battery life or device efficiency. With this architecture, Apple can push the boundaries of mobile AI, embedding sophisticated models into daily user experiences while maintaining security and privacy. |
Privacy-Centric AI Architecture |
Perhaps the most significant development is Apple’s approach to privacy. With Apple Intelligence, users can access private cloud compute for more resource-hungry generative models. The brilliance here is in the architecture: sensitive data stays on the device, and any cloud interaction is end-to-end encrypted, ensuring privacy is maintained without sacrificing performance. This opens up new opportunities for mobile AI, particularly in sectors where data security is paramount, such as healthcare and fintech. |
Transforming Computational Photography |
The iPhone 16 camera system, powered by this generative AI backbone, takes computational photography to a new level. The 48MP fusion camera now uses the same AI capabilities to enhance image quality, but it’s the integration of visual intelligence that stands out. Think of it as real-time inference running within your pocket – instant identification of objects, text, and even actions through the camera interface. The demo, at least, looks pretty cool and believable. |
A Glimpse into the Future of Edge AI |
For ML engineers and AI researchers, the iPhone 16 represents an intriguing case of edge AI deployment. As we move toward a future where models can be fine-tuned and run efficiently on consumer devices, Apple’s hardware-software integration sets a precedent. |
Hearing Aid |
I have to mention what Apple offers now with their AirPods: they introduced end-to-end hearing health experience, which includes the ability to use AirPods Pro 2 as a clinical-grade hearing aid. Bravo and thank you. |
Now, actually looking forward to get a new iPhone 16 Pro and test all new AI stuff myself. |
|
|
Discovering research, made easier with SciSpace |
Imagine having instant access to over 280 million papers, breezing through literature reviews, and even chatting with PDFs to get summaries and insights—all with the help of an AI copilot. SciSpace is here to make research feel a lot less like work. |
Give it a try, and if it feels like the perfect fit, you can get 40% off an annual subscription using code “TP40” or 20% off a monthly plan with code “TP20”. |
|
|
|
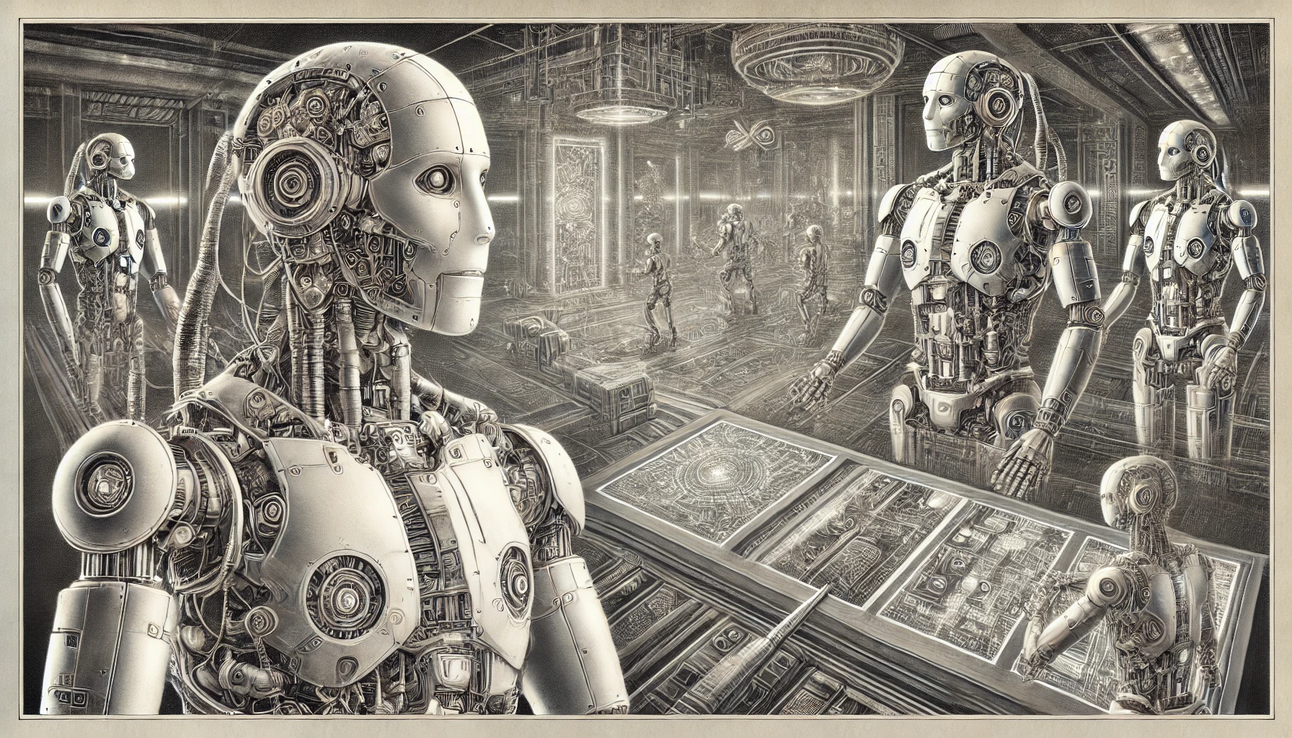 | 7 Sources to Master Robotics | 🔥HOT🔥 Courses and tutorials on how to build robots | www.turingpost.com/p/7-sources-to-master-robotics |
| 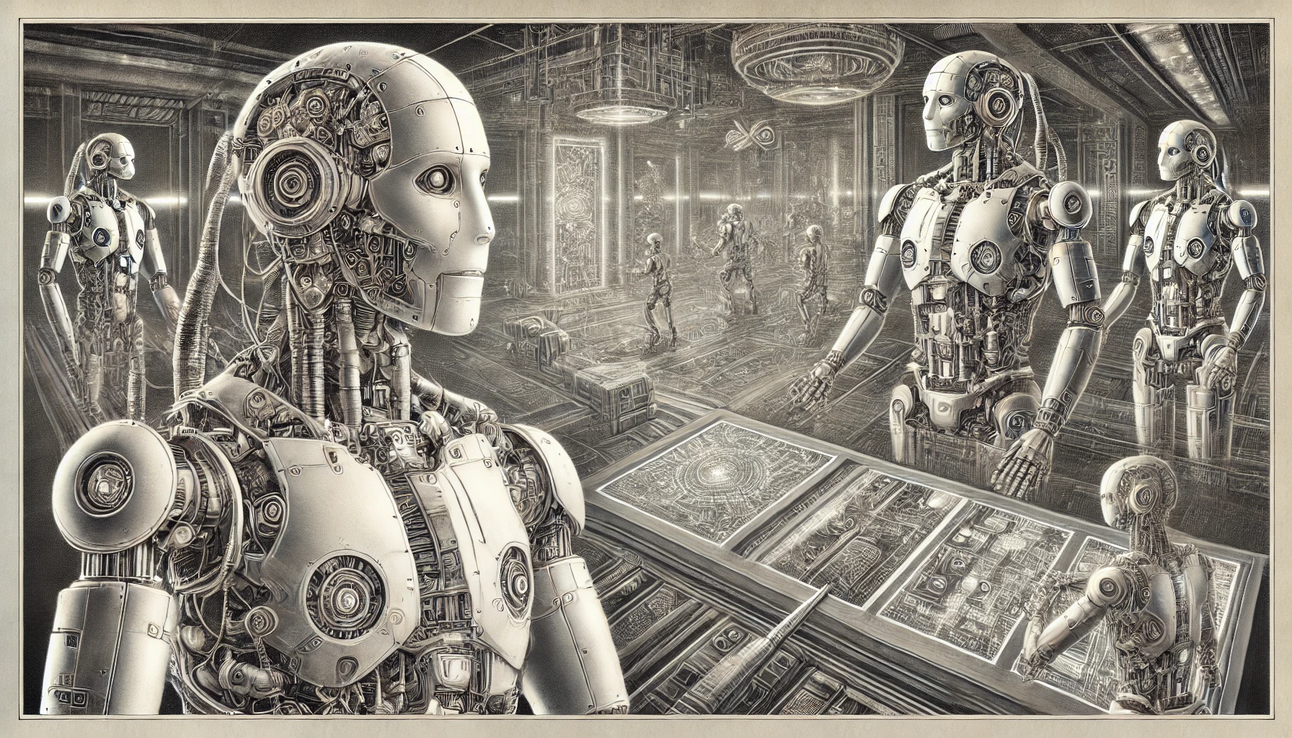 |
|
|
Related to Superintelligence/AGI (new rubric!) |
 | François Chollet @fchollet | 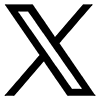 |
| |
The monthly "AGI achieved internally" rumors from OpenAI are like the story of the boy who cried wolf -- except the villagers still show up without fail 30 times in a row, and there were never any wolves living in the province in the first place | | 1:43 PM • Sep 8, 2024 | | | | 1.24K Likes 124 Retweets | 48 Replies |
|
|
|
|
News from The Usual Suspects © |
|
 | clem 🤗 @ClementDelangue | 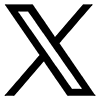 |
| |
As we're seeing more and more everyday, evaluation is one of the most important steps - if not the most important one - in AI. Not only do we need to improve general benchmarking but we should also give every organization to run their own evaluation, aligned with their specific… x.com/i/web/status/1… | | 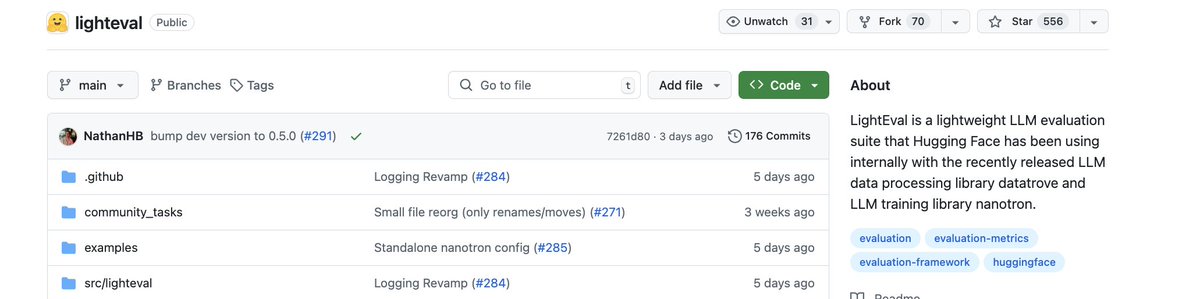 | | 1:31 PM • Sep 9, 2024 | | | | 146 Likes 26 Retweets | 10 Replies |
|
|
|
 | Amjad Masad @amasad | 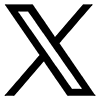 |
| |
AI is incredible at writing code. But that's not enough to create software. You need to set up a dev environment, install packages, configure DB, and, if lucky, deploy. It's time to automate all this. Announcing Replit Agent in early access—available today for subscribers: | | 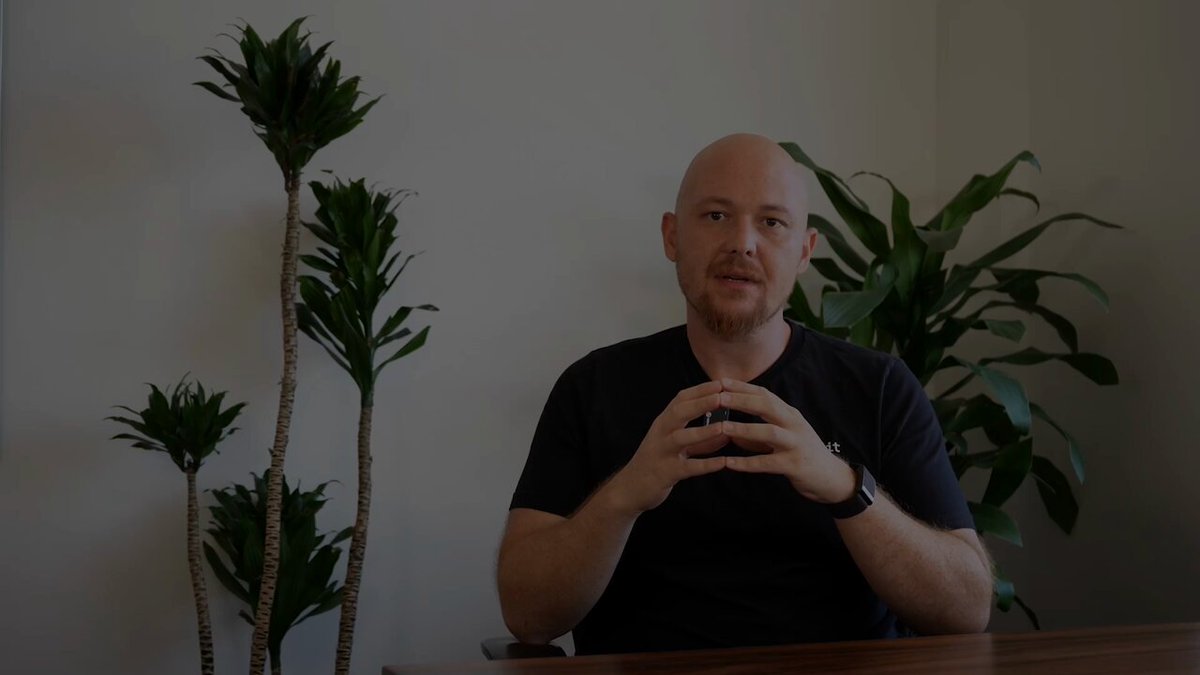 | | 4:27 PM • Sep 5, 2024 | | | | 8.35K Likes 1.11K Retweets | 444 Replies |
|
|
DeepMind’s AlphaProteo Breakthrough DeepMind is simply amazing. Their AlphaProteo is reshaping biotech by designing novel protein binders, crucial for disease-fighting drugs. With successes against SARS-CoV-2 and cancer proteins, AlphaProteo leaps ahead of previous methods, proving that AI is an extremely useful thing.
Salesforce’s xLAM: Actions Speak Louder Salesforce introduces xLAM, a suite of AI models supercharging agent functions and function-calling tasks. With optimized performance on benchmarks like ToolBench, xLAM is built to drive efficiency across sectors, from mobile apps to complex systems. The only sad thing about it is that in Russian it looks like the word ХЛАМ, which means – garbage.
NVIDIA's Narrow Path Nearly half of Nvidia’s $30B Q2 2024 revenue stems from just four heavyweights – Microsoft, Meta, Amazon, and Google. While their AI thirst powers Nvidia's success, such concentration might be dangerous. NVIDIA’s Deep Learning Institute with Dartmouth College launch a free Generative AI Teaching Kit. With modules on LLMs, NLP, and diffusion models, students can now train AI models using NVIDIA’s cloud platform.
Sakana AI: Small Models, Big Dreams Tokyo’s Sakana AI swims upstream with $100M+ in backing, including a splash from NVIDIA. Led by ex-Google Brain David Ha, Sakana's focus on small, energy-efficient AI models aligns with Japan’s ambitions for tech independence. With nature-inspired AI evolution, they're schooling the competition. (*Sakana means fish in Japanese)
SSI: Sutskever’s $1B Bet on Safe AI "Everyone just says 'scaling hypothesis’. Everyone neglects to ask, what are we scaling?" said Ilya. And he himself is now chasing safety. With $1B in funding, his new venture, Safe Superintelligence Inc. (SSI), aims to steer AI towards a future aligned with human values. Early-stage, but already worth $5B. Ilya should feel pretty safe.
|
We are watching/reading: |
|
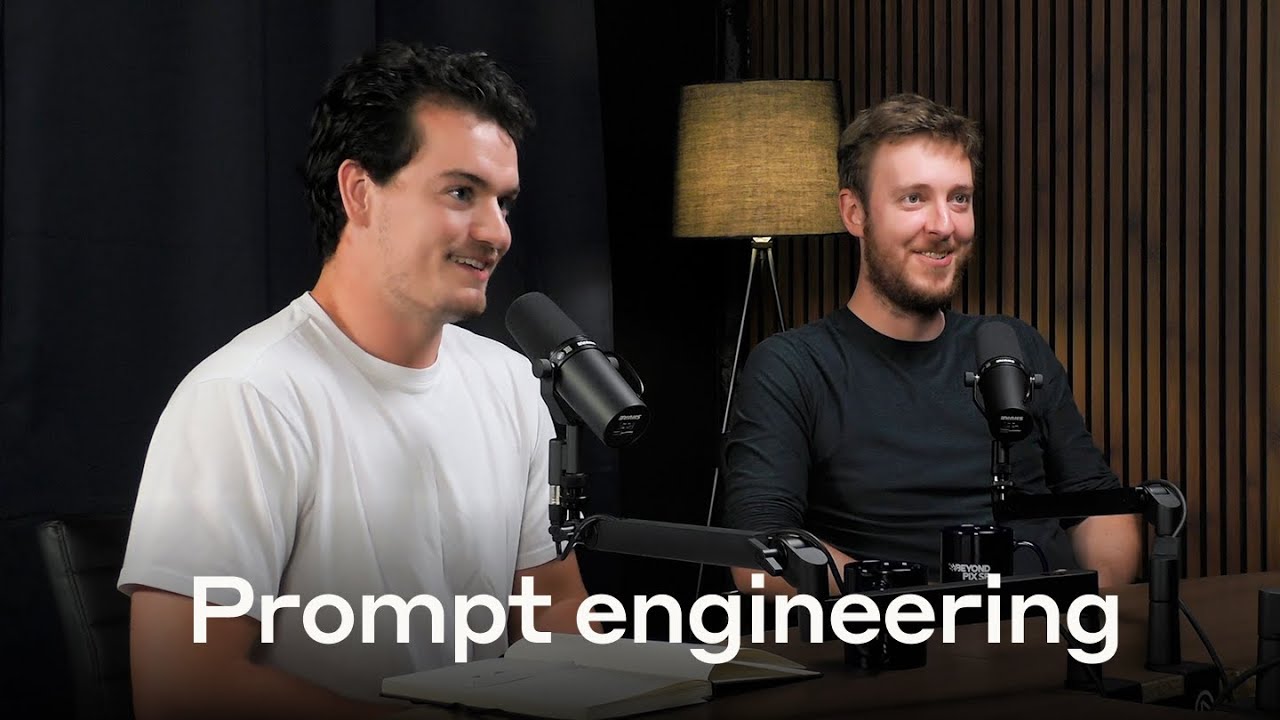 | AI prompt engineering: A deep dive |
|
|
Here AI and data expert and our reader John Thompson summarized 15 main prompt engineering tips from that video. |
The freshest research papers, categorized for your convenience |
Our top (today, it’s all about open-source) |
OLMoE: Open Mixture-of-Experts Language Models Researchers from the Allen Institute developed OLMoE, a sparse Mixture-of-Experts model with 7 billion parameters, but only 1 billion are active per input token. Trained on 5 trillion tokens, the model outperforms larger models like Llama2-13B-Chat and DeepSeekMoE-16B. The team also introduced OLMoE-1B-7B-Instruct, fine-tuned for specific tasks. They release all resources, including model weights, training data, and code, enabling open access to state-of-the-art language model research →read the paper and/or Interconnects’s post
Mini-Omni: Language Models Can Hear, Talk While Thinking in Streaming Researchers from Tsinghua University introduce Mini-Omni, an open-source, end-to-end multimodal model capable of real-time speech interaction. Unlike typical models that rely on text-to-speech systems, Mini-Omni directly generates both speech and text tokens in parallel, minimizing latency. It uses a novel training method, "Any Model Can Talk," to enable rapid speech integration without major architectural changes. Additionally, the VoiceAssistant-400K dataset was created to fine-tune speech output. Mini-Omni's approach significantly enhances real-time conversational AI efficiency →read the paper
InkubaLM: A small language model for low-resource African languages Researchers from Lelapa AI, MBZUAI, McGill University, and University of Pretoria developed InkubaLM, a 0.4 billion parameter multilingual model focused on five African languages: Swahili, Hausa, Yoruba, isiZulu, and isiXhosa. Despite its small size, InkubaLM performs comparably to larger models in tasks like machine translation, question-answering, and sentiment analysis. The model outperforms others in sentiment analysis for Swahili and achieves significant efficiency using custom Flash Attention. Its datasets and model are open-source to advance NLP for low-resource African languages →read the paper
|
Mathematical Reasoning and Scientific Text Understanding |
Building Math Agents with Multi-Turn Iterative Preference Learning proposes a multi-turn learning method to enhance LLMs in mathematical reasoning by integrating external tools like code interpreters, improving performance on math tasks like GSM8K and MATH datasets →read the paper SciLitLLM: How to Adapt LLMs for Scientific Literature Understanding introduces SciLitLLM, an LLM specialized in understanding scientific texts, showing improved performance through continual pre-training and supervised fine-tuning on scientific literature benchmarks →read the paper
|
Code Generation and Data Optimization |
How Do Your Code LLMs Perform? Empowering Code Instruction Tuning with High-Quality Data analyzes data quality in code instruction tuning and proposes a data pruning strategy that improves LLM performance using cleaner datasets →read the paper Arctic-SnowCoder: Demystifying High-Quality Data in Code Pretraining presents a model pre-trained on a high-quality dataset, showing the value of domain-aligned data in improving code generation performance →read the paper
|
AI Agents and Decision-Making |
Agent Q: Advanced Reasoning and Learning for Autonomous AI Agents combines Monte Carlo Tree Search and self-critique to improve autonomous decision-making, significantly boosting success rates in web tasks like e-commerce →read the paper From MOOC to MAIC: Reshaping Online Teaching and Learning through LLM-driven Agents proposes an AI-powered learning platform utilizing LLM-driven agents to enhance online education's scalability and personalization →read the paper
|
Long Context and Citation Generation |
Speculative decoding for high-throughput long-context inference accelerates long-context LLM inference by addressing memory bottlenecks, achieving significant speedups in large-scale LLM operations →read the paper LONGCITE: Enabling LLMs to Generate Fine-Grained Citations in Long-Context QA introduces LongCite to improve citation accuracy and reduce hallucinations in long-context question-answering tasks →read the paper
|
Privacy and Contextualization |
PrivacyLens: Evaluating Privacy Norm Awareness of Language Models in Action introduces a framework for assessing LLM privacy awareness, revealing significant privacy risks during real-world tasks despite privacy-focused prompts →read the paper ContextCite: Attributing Model Generation to Context presents a method to attribute specific outputs of LLMs to their input context, improving statement verification and security →read the paper
|
Robotic Applications and Policy Optimization |
Diffusion Policy Policy Optimization presents a new method for fine-tuning diffusion-based policies in reinforcement learning, excelling in robotic control tasks, particularly those with sparse rewards →read the paper
|
General LLM Theory and Modular Approaches |
Configurable Foundation Models: Building LLMs from a Modular Perspective introduces a modular approach to building LLMs, allowing for task flexibility and scalability by decomposing models into functional modules →read the paper General OCR Theory: Towards OCR-2.0 via a Unified End-to-end Model proposes an integrated, end-to-end OCR model that improves efficiency and accuracy across a range of optical tasks, from text to complex documents →read the paper
|
Please send this newsletter to your colleagues if it can help them enhance their understanding of AI and stay ahead of the curve. You will get a 1-month subscription! |
|
|
|