| | Wow. This week is going to be hot! I’m in Seattle right now to cover Microsoft Build and bring you insights from Kevin Scott, Microsoft's CTO. | Since it's all very exciting and reportage is a completely different thing than just a pure analysis based on 150+ newsletters and media well-read, we are changing our usual schedule. This week, you will receive two FODs: | Today, on Monday, we will cover the news from Google I/O and their moat against OpenAI. Tomorrow, on Tuesday, fresh and hot, right after our conversation with Kevin Scott, we will send you what caught our attention from Microsoft's announcements (they already announced Surface Pro 11, Surface Laptop 7, and Copilot+ PCs powered by Snapdragon X Elite – but there is more to come!)
| Are you also in Seattle? Let me know, maybe we can catch up for a coffee. | If you like Turing Post, consider becoming a paid subscriber. You’ll immediately get full access to all our articles, investigations, and tech series → | |
|
| Google’s moat against OpenAI | Last week saw two big tech events: the OpenAI Spring updates and Google I/O. We covered OpenAI’s impressive presentation of GPT-4o and thought OpenAI would “rest on their laurels,” but by the end of the week, a few notable resignations occurred. Ilya Sutskever, Jan Leike, and Evan Morikawa left the company. When many scientific personnel depart, it often indicates a shift in favor of product-oriented priorities, which is concerning, considering OpenAI’s goal to achieve not-fully specified AGI. It’s sad that while delivering so much, they are also notable for frequent drama and reactive damage control. | This brings us to Google. After their updates last week during Google I/O, some observers noted that Google, which hasn't partnered with any foundation model builders (such as OpenAI, Anthropic, Mistral, etc.), is catching up quickly. Considering the turmoil at OpenAI, it’s safe to say that Google’s moat – initially perceived as a disadvantage – lies in their size and history. Google is a large, established tech company with diversified revenue streams, demonstrating financial stability and consistent growth. It’s basically drama-free. Sundar Pichai is well-paced and plays a very long-term game. He and Google might seem to move slower at first, but they have tremendous ML talent, developed infrastructure, and business applications for their AI. Google's steady, methodical approach could prove to be more resilient in the long run. | But, there are different opinions as well. Stratechery argues that weaknesses emerge in Google's innovation pipeline outside its core competencies. The disappointment highlighted during the Google I/O keynote, for instance, stems from what appears to be a series of underdeveloped new products that do not yet match the transformative impact of its existing technologies. Additionally, many of Google's ambitious projects, such as AI Agents and Project Astra, are still at a conceptual stage without immediate practical applications, leading to perceptions of them as vaporware. These initiatives show potential but also reveal a gap between Google's visionary presentations and their current practical implementations. This gap may affect Google's ability to maintain its innovative edge against rapidly evolving competitors in the AI space. | | Google I/O 2024 was, of course, a showcase of the company's deepening commitment to AI. Here are highlights: | Gemini Enhancements & Integrations: | Google's Gemini model was taking center stage. An incredible upgrade is the doubling of Gemini 1.5 Pro's context window from 1 million to 2 million tokens, enhancing its ability to understand and respond to complex queries. Google's latest language model is not only getting faster and more capable but is also being integrated across various Google products (such as Gmail, Drive, Docs, etc).
| Generative AI Innovations: | Beyond Gemini, Google introduced PaliGemma, a powerful open vision-language model inspired by PaLI-3. PaliGemma combines the SigLIP vision model and Gemma language model for class-leading performance in tasks like image captioning, visual question answering, and object detection. And unveiled Gemma 2, a next-generation AI model with 27 billion parameters, offering class-leading performance at half the size of comparable models like Llama 3 70B. And set a waitlist for Imagen-3, their highest quality text-to-image model. Google also presented Project Astra, an ambitious endeavor to create a multimodal AI assistant that “can process multimodal information, understand the context you're in, and respond naturally in conversation.” Another notable reveal was Veo, a GenAI model capable of producing 1080p videos from text, image, or video prompts, opening new creative possibilities. ElevenLabs immediately gave it a try:
|  | ElevenLabs @elevenlabsio | 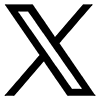 |
| |
We loved what we saw with Veo at Google I/O so we turned it into a music video using our AI generated sound effects and music. Take a listen. | 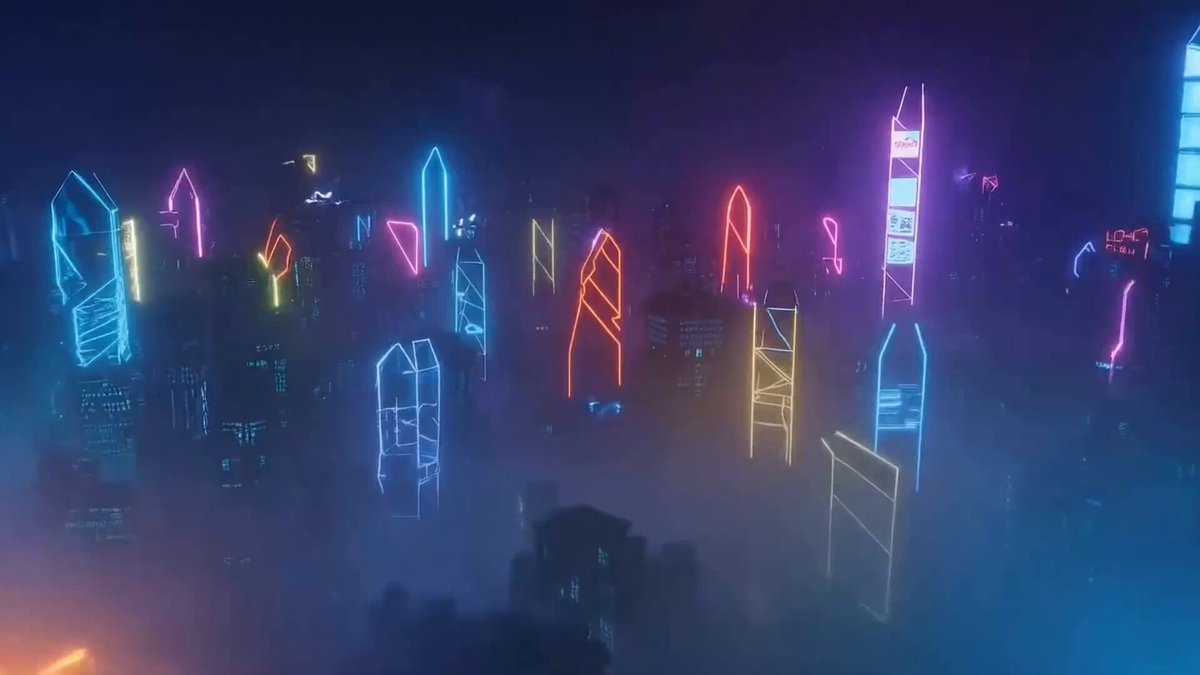 | | May 15, 2024 | | | | 243 Likes 24 Retweets 21 Replies |
|
| | Search & Information Access Improvements | Very cool feature: “Ask Photos”, powered by Gemini, enables users to query their photo libraries conversationally. Google Chrome is also getting smarter with the integration of Gemini Nano, facilitating text generation within the browser. Google Search is receiving an AI overhaul with "AI Overviews," summarizing information from the web, and a new "Circle to Search" feature for solving math problems. Finally, Google's SynthID, an AI watermarking tool, is being upgraded to detect AI-generated videos and images. Google Lens received a significant upgrade, allowing users to search using video recordings.
| Hardware | Everybody tries to announce something about compute. At Google I/O 2024, Google unveiled Trillium, its sixth-generation TPU, offering a 4.7x increase in compute performance per chip, double the HBM and ICI bandwidth, and 67% greater energy efficiency. Featuring third-generation SparseCore, Trillium supports large-scale AI models like Gemini 1.5 Flash and Imagen 3. These TPUs can scale to hundreds of pods, forming supercomputers, and enhance AI workloads, supporting frameworks like JAX and PyTorch/XLA.
| Overall, Google I/O 2024 underscored the company's focus on making AI more accessible, powerful, and integrated into everyday tools and experiences. The event set the stage for a future where AI plays an even more significant role in how we interact with technology and information. | Google I/O Keynote: | 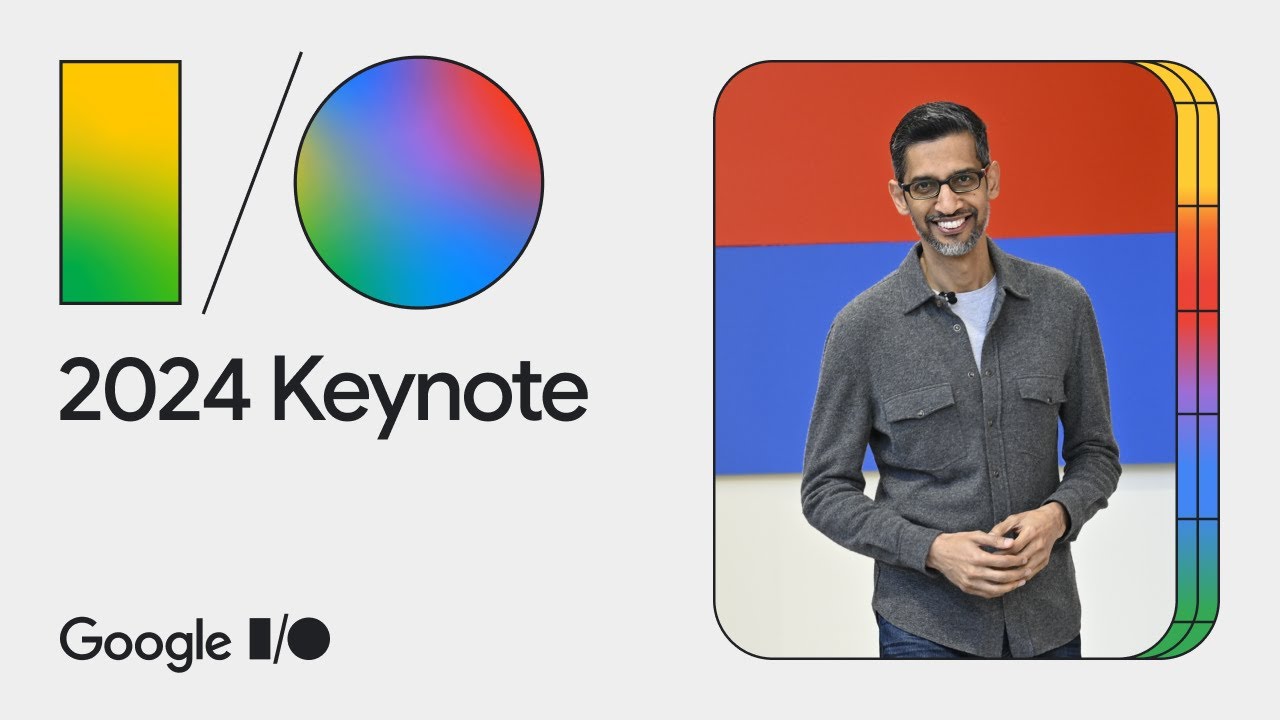 | Google Keynote (Google I/O ‘24) |
|
| |
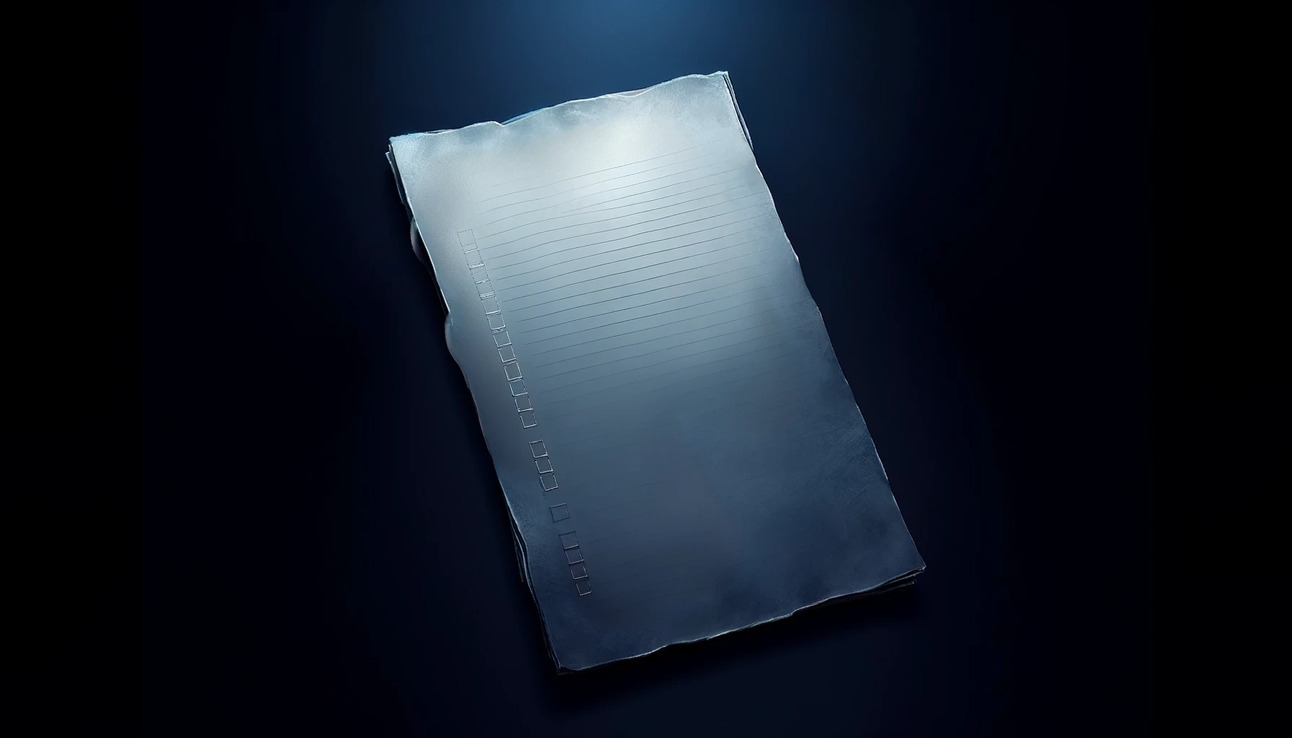 | The Mysterious AI Reading List: Ilya Sutskever's Recommendations | A List Everyone Talks About, But No One Has Ever Seen | www.turingpost.com/p/ilya-sutskever-reading-list |
| 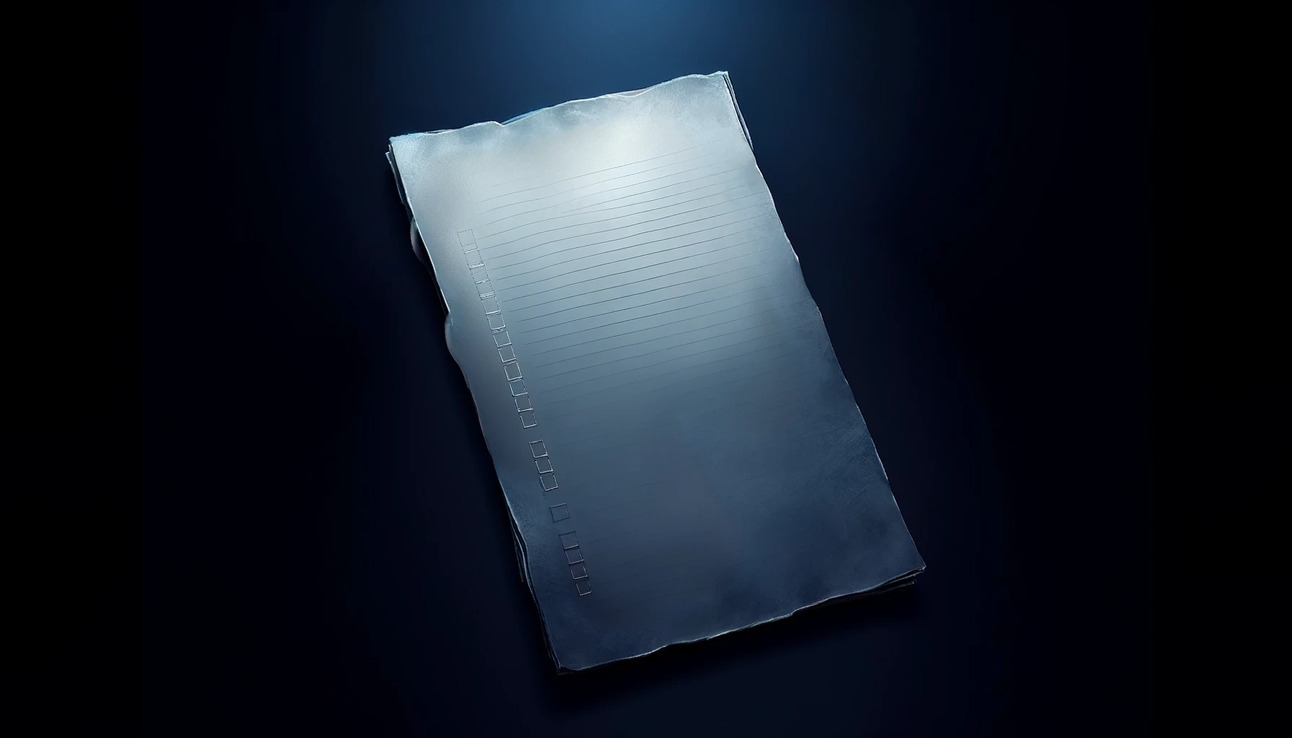 |
|
| | News from The Usual Suspects © | Microsoft’s turn to shine | Microsoft Build kicks off tomorrow, May 21-23, but they has already announced Surface Pro 11, Surface Laptop 7, and Copilot+ PCs powered by Snapdragon X Elite. These processors are expected to boost AI performance, potentially surpassing the M3 MacBook Air. New Copilot features include Recall search for comprehensive file history retrieval, Cocreator for image generation, and AI-generated in-game hints for Xbox Game Pass. Stay tuned for tomorrow!
| Hugging Face’s cool launches | HF introduced ZeroGPU – a significant step forward in democratizing AI technology by providing shared GPU infrastructure. Independent and academic AI developers often lack the resources available to big tech companies. ZeroGPU allows users to run AI demos efficiently on shared GPUs without bearing high compute costs, offering $10M of free GPUs to support this initiative. The infrastructure uses Nvidia A100 GPU devices and operates more energy-efficiently by dynamically allocating GPU resources, which can host multiple spaces simultaneously. HF launched Transformers Agents 2.0, an updated framework for creating agents that solve complex tasks by iterating based on past observations.
| Some good news from OpenAI |  | OpenAI @OpenAI | 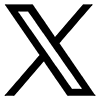 |
| |
We're rolling out interactive tables and charts along with the ability to add files directly from Google Drive and Microsoft OneDrive into ChatGPT. Available to ChatGPT Plus, Team, and Enterprise users over the coming weeks. |  | openai.com/index/improvem… Improvements to data analysis in ChatGPT Improvements to data analysis in ChatGPT Interact with tables and charts and add files directly from Google Drive and Microsoft OneDrive. |
|
| | May 16, 2024 | | | | 8.53K Likes 1.27K Retweets 360 Replies |
|
| In other newsletters: | | BTW, you might also like this episode about ImageNet. Read it here, It’s free |  | Fei-Fei Li @drfeifei | 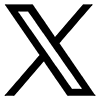 |
| |
This is a really good summary of how the breakthroughs in Neural Network (AlexNet), Big Data (ImageNet) and GPUs led to the birth of modern AI and computer vision. Thank you @Kseniase_ and your @TheTuringPost ! | Ksenia Se @Kseniase_ This is how ImageNet, a massive dataset opened for collaboration, started to influence the deep learning revolution. But it wasn't just ImageNet. Check out other revolutionary AI developments like AlexNet and GPUs in my article at @TheTuringPost! 7/7 |
| | May 20, 2024 | | | | 237 Likes 56 Retweets 5 Replies |
|
| The freshest research papers, categorized for your convenience | AI Model Innovations and Performance Enhancements | DeepSeek-V2: A Strong, Economical, and Efficient Mixture-of-Experts Language Model: Develops a high-performance, cost-effective Mixture-of-Experts model that showcases significant enhancements in training cost reduction and computational efficiency →read the paper Characterizing the Accuracy - Efficiency Trade-off of Low-rank Decomposition in Language Models: Examines Tucker decomposition to optimize the balance between model size reduction and performance retention in language models →read the paper SambaNova SN40L: Scaling the AI Memory Wall with Dataflow and Composition of Experts: Addresses the memory wall challenge in AI accelerators with a novel architecture that leverages a Composition of Experts for improved performance →read the paper Many-Shot In-Context Learning in Multimodal Foundation Models: Explores the enhancement of in-context learning capabilities in multimodal foundation models using many-shot learning, demonstrating improvements in performance and efficiency across diverse datasets →read the paper MambaOut: Do We Really Need Mamba for Vision?: Evaluates the necessity of Mamba's state space model for vision tasks, demonstrating that while it may not be essential for image classification, it holds potential benefits for more complex tasks like object detection and segmentation →read the paper
| Security and Ethical Considerations in AI | | Benchmarks and Evaluations in AI Research | Plot2Code: A Comprehensive Benchmark for Evaluating Multi-modal Large Language Models in Code Generation from Scientific Plots: Creates a benchmark for assessing LLMs' ability to interpret and code from scientific plot visuals →read the paper MS MARCO Web Search: A Large-scale Information-rich Web Dataset with Millions of Real Click Labels: Introduces a substantial web dataset to support advancements in AI and large-scale information retrieval →read the paper
| Strategic Frameworks and Theoretical Advances in AI | RLHF Workflow: From Reward Modeling to Online RLHF: Presents a comprehensive strategy for implementing online reinforcement learning from human feedback, documenting performance gains over offline methods →read the paper Beyond Scaling Laws: Understanding Transformer Performance with Associative Memory: Develops a theoretical approach using associative memory to analyze and explain transformer model behaviors →read the paper Position: Leverage Foundational Models for Black-Box Optimization: Advocates integrating LLMs with black-box optimization processes, proposing new ways to leverage AI for complex decision-making →read the paper
| Research Surveys and Comparative Studies | A Survey on RAG Meets LLMs: Towards Retrieval-Augmented Large Language Models: Surveys developments in retrieval-augmented generation techniques, analyzing their impact on enhancing LLM performance and mitigating limitations →read the paper Understanding the performance gap between online and offline alignment algorithms: Delves into the disparities between online and offline alignment methods in reinforcement learning, elucidating their strengths and weaknesses →read the paper
| If you decide to becoming a Premium subscriber, you can expense this subscription through your company. Please also send this newsletter to your colleagues if it can help them enhance their understanding of AI and stay ahead of the curve. |
|
| | Thank you for reading! We appreciate you. 🤍 | How was today's FOD?Please give us some constructive feedback | | |
|